Introduction
Machine learning (ML) technologies are advancing rapidly, particularly in the field of medical imaging. Over the past decade, there has been significant growth in the application of ML in medical imaging for detection, diagnosis, triaging, and clinical decision support of various conditions. The ultimate aim of these developments is to bring safe and effective technologies to clinical settings for the benefit of patients. Regulatory oversight is essential in this process, ensuring that these technologies meet safety, efficacy, and quality standards.
The Center for Devices and Radiological Health (CDRH) at the FDA is responsible for ensuring that patients and healthcare providers have timely and continued access to safe, effective, and high-quality medical devices. Regulatory science plays a crucial role in this mission by developing new tools, standards, and methods to assess the safety, efficacy, quality, and performance of FDA-regulated products.
Imaging Device Regulation Overview
We won’t go into details on the basics of FDA medical device classification [1]. Most medical image processing devices are classified as Class II devices. Software-only devices or Software as a Medical Device (SaMD) that are intended for image processing are usually classified as picture archiving and communications systems, or PACS, (see 21 CFR 892.2050). In 2021, the FDA updated the name of regulation 21 CFR 892.2050 to “medical image management and processing system.” Currently, there are no mandatory special controls for software-only devices under this regulation. The primary resource for understanding the performance data requirements for such devices is the guidance document on the 510(k) Substantial Equivalence [2]. For devices focused on quantitative imaging, understanding what’s expected for performance requirements can be in the FDA guidance for quantitative imaging [3].
On the other hand, devices classified under the De Novo pathway have “Special controls'' that manufacturers must adhere to. Devices classified through the De Novo pathway include special controls that outline requirements for these devices - including clinical and non-clinical performance requirements. Devices, including those implementing machine learning, must comply with the special controls. The classification and special controls are published in the Federal Register and appear in the Electronic Code of Federal Regulations (eCFR). One can also find these outlined in the original De Novo Reclassification Order. You can read more about the De Novo process in the FDA guidance document [4] and you can search for De Novo granted devices in the De Novo database [5].
Examples of devices classified via the De Novo pathway include:
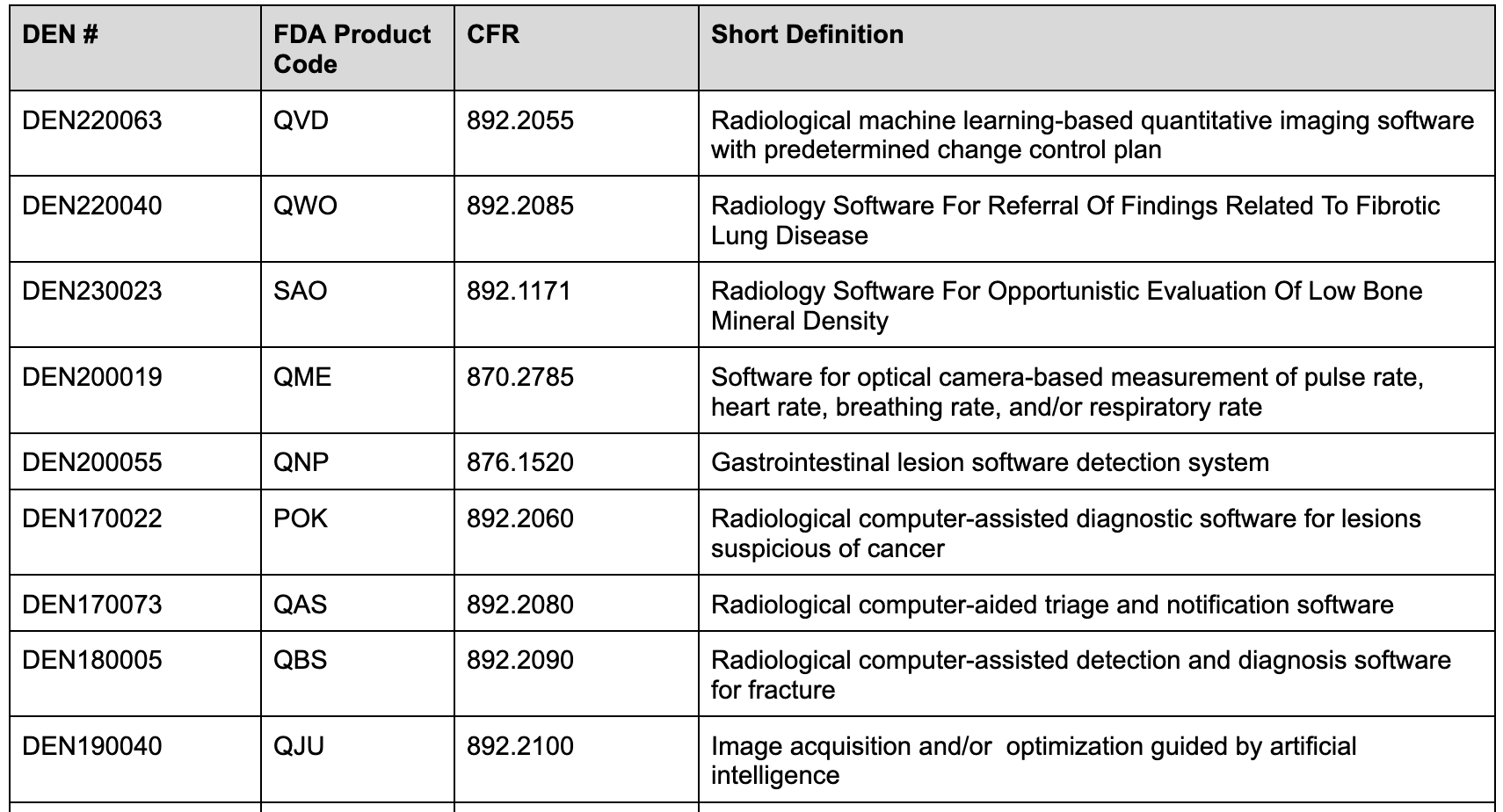
Special controls are designed to mitigate health risks associated with these devices. Many of these relate directly to elements associated with machine learning-based software for detection or diagnostics, including performance testing and characterization requirements. For new devices, the FDA generally requires both standalone and clinical testing to demonstrate diagnostic accuracy and/or improvement. Devices implementing machine learning algorithms for estimating other physiological characteristics may also need standalone and clinical testing. Examples of devices only needing standalone testing are machine algorithms focused on segmentation and or quantification tasks.
Least Burdensome Approach & Real-World Data
The CDRH is required by law to consider the least burdensome approach to regulatory requirements [6]. This includes considering alternative methods, data sources, real-world evidence, nonclinical data, and other means to meet regulatory requirements. The FDA encourages innovative approaches to device design and regulatory compliance. They take a risk-benefit approach to novel devices and those with different technological characteristics.
Additionally, the FDA evaluates Real-World Data (RWD) to determine if it is sufficient to be used in regulatory decision making for medical devices and provides expanded recommendations for sponsors collecting RWD [7].
Pre-Submission Meetings
CDRH offers opportunities for developers to request feedback and meet with FDA staff before making a premarket submission. These interactions focus on specific devices and questions related to planned submissions, including non-clinical testing protocols, proposed labeling, regulatory pathways, and clinical study design and performance criteria. Read more about the FDA Q-sub program [8] and pre-submission meetings to understand when is the best time to engage with the FDA. Generally, we believe it’s most useful when you have a clear product description, intended use and indications for use, and a high-level performance test plan and protocol they can review.
Product Life Cycle Considerations
Device developers should be aware of regulatory requirements throughout the product life cycle, including investigational device requirements (e.g., 21 CFR 812), premarket requirements, postmarket requirements (e.g., 21 CFR 820), and surveillance requirements. While this blog post focuses on premarket and performance assessment, it is important to consider regulatory requirements throughout the device life cycle. The FDA has provided guidance on premarket considerations for device software functions [9]. Another important consideration throughout the development of devices implementing machine learning algorithms are Good Machine Learning Practices (GMLP) [10] and risks associated with AI/ML technologies. To get a better sense of what AI/ML risks to consider, we encourage you to purchase the AAMI TIR34971:2023 consensus report [14].
AI Risks
Taking a deeper dive into risks, risks from AI-based technology is different from traditional software and can be bigger than an enterprise, span organizations, and lead to societal impacts. AI systems also bring a set of risks that are not comprehensively addressed by current risk frameworks and approaches. Some AI system features that present risks also can be beneficial. Identifying contextual factors will assist in determining the level of risk and potential management efforts.
Compared to traditional software, AI-specific risks that are new or increased include the following:
- The data used for building an AI system may not be a true or appropriate representation of the context or intended use of the AI system, and the ground truth may either not exist or not be available. Additionally, harmful bias and other data quality issues can affect AI system trustworthiness, which could lead to negative impacts.
- AI system dependency and reliance on data for training tasks, combined with increased volume and complexity typically associated with such data.
- Intentional or unintentional changes during training may fundamentally alter AI system performance.
- Datasets used to train AI systems may become detached from their original and intended context or may become stale or outdated relative to deployment context.
- AI system scale and complexity (many systems contain billions or even trillions of decision points) housed within more traditional software applications.
- Use of pre-trained models that can advance research and improve performance can also increase levels of statistical uncertainty and cause issues with bias management, scientific validity, and reproducibility.
- Higher degree of difficulty in predicting failure modes for emergent properties of large-scale pre-trained models.
- Privacy risk due to enhanced data aggregation capability for AI systems.
- AI systems may require more frequent maintenance and triggers for conducting corrective maintenance due to data, model, or concept drift.
- Increased opacity and concerns about reproducibility.
- Underdeveloped software testing standards and inability to document AI-based practices to the standard expected of traditionally engineered software for all but the simplest of cases.
- Difficulty in performing regular AI-based software testing, or determining what to test, since AI systems are not subject to the same controls as traditional code development.
- Computational costs for developing AI systems and their impact on the environment and planet.
- Inability to predict or detect the side effects of AI-based systems beyond statistical measures.
AI Transparency
To answer a demand for increased transparency around machine learning based device functions, and to more fully satisfy the requirement that 510(k) summaries provide “an understanding of the basis for a determination of substantial equivalence”, as part of your premarket submission, the FDA will need to understand your training methodologies, what your training dataset looked like, as well as have a detailed description of what you model architecture is with a comprehensive description of the various components of the architecture. Additionally, in your product labeling, you will need to summarize your training methodology, the training dataset, and your performance testing methodology and results.
Predetermined Change Control Plans (PCCP)
A device developer implementing machine learning algorithms can also include a Predetermined Chance Control Plan, or PCCP, in their premarket submissions. A PCCP allows a developer to include detailed descriptions and test plans for future algorithm modifications. Acceptance of such a plan would mean the developer would not need to seek FDA review for a modification that was covered and cleared as part of the original device clearance. Read more about PCCPs and how you can leverage them in our recent post "What is an FDA PCCP" [11].
Cybersecurity
In most cases, machine learning algorithms - and how users interact with them - are hosted on a secure cloud service and can be/are integrated into any radiology visualization platform. In these cases, it’s important to consider applicable cybersecurity requirements. The FDA has provided various guidances both premarket [12] and postmarket [13]. Note that in 2023 they recognized the ANSI/AAMI SW96 cybersecurity standard that takes into account the aforementioned guidances.
Conclusion
In conclusion, there are over 850 AI/ML devices now cleared by the FDA and over 300 Radiology devices leveraging AI/ML cleared by the FDA as of this writing. The integration of AI and ML in Radiology represents a significant leap forward in medical imaging, offering the potential for more accurate, efficient, and accessible healthcare solutions. Navigating the FDA regulatory pathway is crucial to ensure these innovative technologies meet rigorous safety and effectiveness standards. The evolving landscape of regulatory science, with initiatives like the De Novo classification pathway and the Least Burdensome Approach, supports the development and approval of AI/ML devices while maintaining a high standard of patient care.
Real-world data, pre-submission meetings, and cybersecurity considerations further underscore the complexity and importance of a thorough regulatory process. As AI/ML technologies continue to advance, so too must our approaches to risk management and transparency to maintain trust and efficacy in clinical applications.
In conclusion, the journey from development to market for AI/ML radiology devices is challenging yet rewarding. By adhering to regulatory guidelines and embracing innovative compliance strategies, developers can contribute to a future where advanced medical imaging technologies significantly improve patient outcomes. The commitment to safety, transparency, and continual improvement will be key in harnessing the full potential of AI/ML in healthcare.
Work With Us
If you're looking for more hands-on help navigating the FDA pathways and frameworks, don't hesitate to reach out to schedule a call. You can reach us at info@cosmhq.com
Resources
[1] Cosm Blog - FDA Medical Device Regulations Overview - https://bit.ly/3Vkvaoz
[2] FDA Guidance - The 510(k) Prorgram: Evaluating Substantial Equivalence - https://www.fda.gov/regulatory-information/search-fda-guidance-documents/510k-program-evaluating-substantial-equivalence-premarket-notifications-510k
[3] FDA Guidance - Technical Performance Assessment of Quantitative Imaging in Radiological Device Premarket Submissions - https://www.fda.gov/media/123271/download
[4] FDA Guidance - De Novo Classification Process - https://www.fda.gov/regulatory-information/search-fda-guidance-documents/de-novo-classification-process-evaluation-automatic-class-iii-designation
[5] FDA De Novo database - https://www.accessdata.fda.gov/scripts/cdrh/cfdocs/cfPMN/denovo.cfm
[6] FDA Least Burdensome Provisions - https://www.fda.gov/regulatory-information/search-fda-guidance-documents/least-burdensome-provisions-concept-and-principles
[7] FDA Draft Guidance - Use of Real-World Evidence to Support Regulatory Decision-Making in Medical Devices https://www.fda.gov/regulatory-information/search-fda-guidance-documents/draft-use-real-world-evidence-support-regulatory-decision-making-medical-devices
[8] FDA Guidance - Requests for Feedback and Meetings for Medical Device Submissions: The Q-Submission Program - https://www.fda.gov/regulatory-information/search-fda-guidance-documents/requests-feedback-and-meetings-medical-device-submissions-q-submission-program-draft-guidance
[9] FDA Guidance - Content of Premarket Submissions for Device Software Functions - https://www.fda.gov/regulatory-information/search-fda-guidance-documents/content-premarket-submissions-device-software-functions
[10] FDA Guidance - Good Machine Learning Practices (GMLP) - https://www.fda.gov/medical-devices/software-medical-device-samd/good-machine-learning-practice-medical-device-development-guiding-principles
[11] Cosm Blog - Predetermined Change Control Plans (PCCP) - https://bit.ly/4aIfeRu
[12] FDA Guidance - Cybersecurity in Medical Devices: Quality System Considerations and Content of Premarket Submissions - https://www.fda.gov/regulatory-information/search-fda-guidance-documents/cybersecurity-medical-devices-quality-system-considerations-and-content-premarket-submissions
[13] FDA Guidance - Postmarket Management of Cybersecurity in Medical Devices - https://www.fda.gov/regulatory-information/search-fda-guidance-documents/postmarket-management-cybersecurity-medical-devices
[14] AAMI CR34971:2022 AAMI Consensus Report - Guidance on the Application of ISO 14971 to Artificial Intelligence and Machine Learning - https://webstore.ansi.org/standards/aami/aamicr349712022 Note that the FDA has recognized CR34971:2022 but there is also TIR34971:2023 - https://store.aami.org/s/store#/store/browse/detail/a154U000006SQauQAG Notably, this is a joint effort between AAMI and BSI and the content of the TIR is substantively the same as the CR content, with only minor spelling and formatting differences between the U.S. and British versions.
Image Source: Created with assistance from ChatGPT, powered by OpenAI
Disclaimer - https://www.cosmhq.com/disclaimer